I am sorry for this naive question, but is critically related to a basic step in RNA seq analyses. I am trying different normalization approach mainly CPM and TMM Assuming the following:
- my raw count is named as 'counts.keep'
- I am using a DGElist called 'dgeObj'
First I tried to get the log2 of just the raw count and created an object called M
M<- log2(counts.keep)
Second, I created the CPM of the log2 using the dgeObj, this object is not TMM normalized ... This creating logcounts (I assume this is the CPM of the log2 count)
logcounts <- cpm(dgeObj,log=TRUE)
Third, I tried to get the TMM normalized count, and here is my question. I used this code
dgeObj <- calcNormFactors(dgeObj)
logCPM <- cpm(dgeObj, log = TRUE)
I first make TMM normalization on the dgeObj, then used cpm function with log =TRUE on this dgeObj
What the cpm actually is doing here? is it making a cpm on top of the TMM normalized reads ? or only doing TMM ?
In another word, I would like to know if I plot the logCPM object, will this be only the TMM normalization or CPM + TMM normalization. So my confusion is can one apply CPM on top on TMM approach or normalize using only one of them to assess the normalization ?
Thanks
Thanks a lot. I actually understand this. My question is what happens in the resultant counts (and hence in their normalization) when using cpm on a DGEList object that have norm.factors ,,, compared to using cpm on a DGEList with no factor (factor = 1) ? I am asking this because some peoples think in the first case it is applying cpm on top of factor-corrected counts (what we might call as TMM normalization). I observed when ploting log2, cpm, cpm + TMM, that normalization is gettting better . see image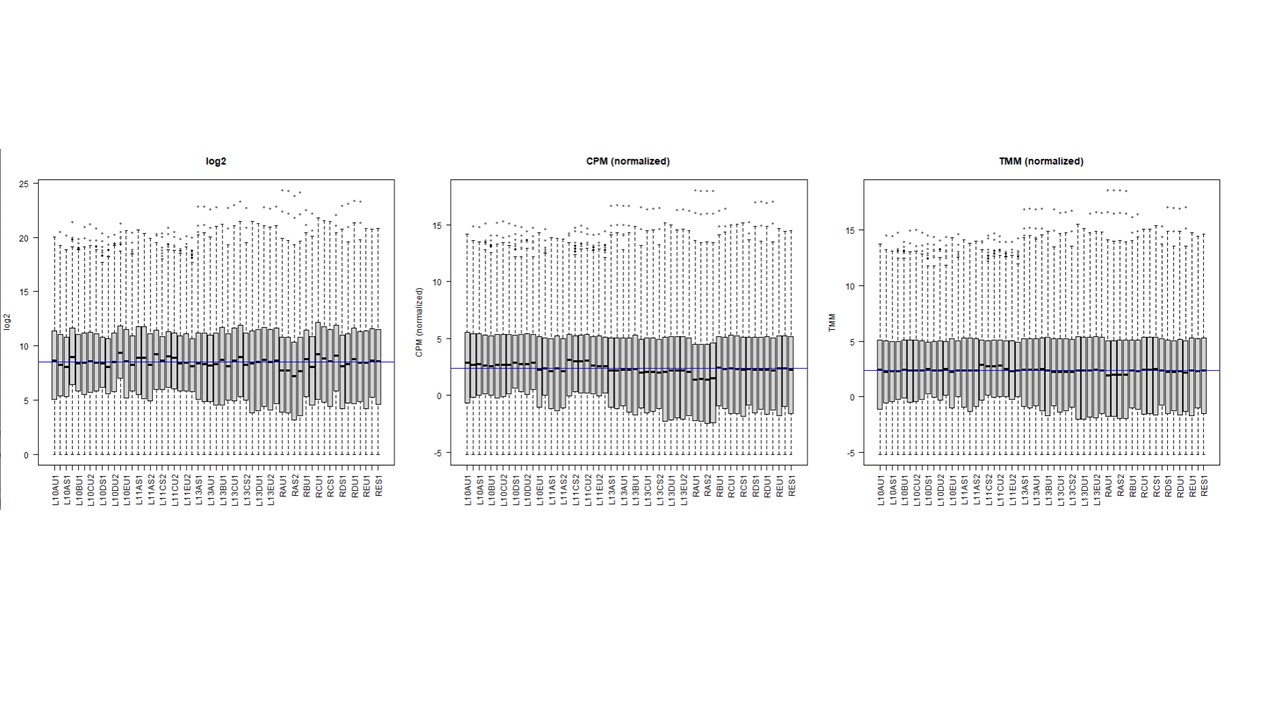
Also ca you advice on any other way to test if certain normalization is performing better that other ?
Thanks
I just explained how it works, and you told me you understand all that, but then ask the same question all over again? Perhaps you should re-read my post and the help page I pointed you towards.
Also there is no way to 'test if a certain normalization is performing better' because we don't know the underlying truth. We make assumptions that seem reasonable and then go forward with the analysis. And when you present your results you say what you did, and perhaps why you think it was a reasonable thing to do.
Sorry for misunderstanding. What I meant was that there is always two option of using cpm on DGElist as shown in my above codes: Option 1: make cpm on DGE list with the normalization factor being 1 (so before making: dgeObj <- calcNormFactors(dgeObj) Option 2: make cpm on DGElist after performing TMM, when we have normalization factors shown in the sample slot of the DGElist (so after applying this : dgeObj <- calcNormFactors(dgeObj))
So my question was what is the difference in terms of the resultant counts between both options ? and when visualizing that ?. in the boxplot shown above, the 2nd one is when option 1 applies, and the 3rd one is when option 2 applies. I could observe that with cpm on a TMM normalized subjects it is better normalized (so the middle blue median line is almost similar to the black median line of all libraries)... For me the differences between 2 and 3 rd box plot indicate difference underlying counts >> am I right ?
calcNormFactors
normalizes the library sizes.cpm
divides counts by library sizes.Running
cpm
aftercalcNormFactors
uses normalized library sizes. Runningcpm
beforecalcNormFactors
uses unnormalized library sizes. Obviously the former is better than the latter. Why would you normalize the library sizes but then ignore the normalization? That would just make no sense at all.