I am analysing single cell seq data from Maynard et al. and I am getting strange log2 fold change values for a cluster of genes when calculated with DESeq2. When I manually calculate the log fold change this doesnt happen, so I cant understand what is causing this. Using code on another help forum I have plotted the log fold change values so you can see the cluster I am talking about (there are also a couple of genes in the positive axis). There seems to be a strange gap with no fold change values coming out between 10 and 20 in both positive and negative directions.
#inputting data and running DESeq2 - DataS1S5 flow is from a csv of raw counts data with samples and counts for each gene
#and coldata contains the conditions to assign each sample
dds <- DESeqDataSetFromMatrix(countData = DataS1S5Flow, colData = coldata, design = ~condition)
dds <- DESeq(dds)
res <- results(dds)
> head(res)
log2 fold change (MLE): condition S5 vs S1
Wald test p-value: condition S5 vs S1
DataFrame with 6 rows and 6 columns
baseMean log2FoldChange lfcSE stat pvalue padj
<numeric> <numeric> <numeric> <numeric> <numeric> <numeric>
A1BG 5.47265e+00 -2.728897 1.64464 -1.659266 0.0970623 0.468531
A1BG-AS1 2.40010e-01 -1.610200 4.16164 -0.386915 0.6988191 NA
A1CF 0.00000e+00 NA NA NA NA NA
A2M 2.14842e+03 0.498344 1.11919 0.445270 0.6561244 0.937951
A2M-AS1 8.55214e-02 1.133983 4.15238 0.273092 0.7847823 NA
A2ML1 0.00000e+00 NA NA NA NA NA
#comparing fold changes:
##DESeq analysis
FC_DESeq <- res$log2FoldChange
#Export the median ratio normalized matrix
cts_norm <- counts(dds, normalized = T)
#Fold change calculated from the normalized matrix
FC_MLE <- apply(cts_norm, 1, function(x)
log2(mean(x[coldata$condition == "S5"])/mean(x[coldata$condition == "S1"])))
plot(FC_DESeq, FC_MLE)
abline(0, 1)
sessionInfo( )
R version 4.0.3 (2020-10-10)
Platform: x86_64-w64-mingw32/x64 (64-bit)
Running under: Windows 10 x64 (build 19044)
Matrix products: default
locale:
[1] LC_COLLATE=English_United Kingdom.1252 LC_CTYPE=English_United Kingdom.1252 LC_MONETARY=English_United Kingdom.1252
[4] LC_NUMERIC=C LC_TIME=English_United Kingdom.1252
attached base packages:
[1] parallel stats4 stats graphics grDevices utils datasets methods base
other attached packages:
[1] PCAtools_2.2.0 pheatmap_1.0.12 ggpubr_0.4.0 rstatix_0.7.0
[5] Rmisc_1.5.1 plyr_1.8.8 lattice_0.20-41 EnhancedVolcano_1.13.2
[9] ggrepel_0.9.1 DESeq2_1.30.1 SummarizedExperiment_1.20.0 Biobase_2.50.0
[13] MatrixGenerics_1.2.1 matrixStats_0.63.0 GenomicRanges_1.42.0 GenomeInfoDb_1.26.7
[17] IRanges_2.24.1 S4Vectors_0.28.1 BiocGenerics_0.36.1 forcats_0.5.1
[21] stringr_1.4.0 dplyr_1.0.9 purrr_0.3.4 readr_2.1.2
[25] tidyr_1.2.0 tibble_3.1.7 ggplot2_3.3.6 tidyverse_1.3.1
loaded via a namespace (and not attached):
[1] utf8_1.2.2 spatstat.explore_3.0-6 reticulate_1.28 tidyselect_1.1.1 RSQLite_2.2.14
[6] AnnotationDbi_1.52.0 htmlwidgets_1.6.1 grid_4.0.3 BiocParallel_1.24.1 Rtsne_0.16
[11] munsell_0.5.0 codetools_0.2-16 ica_1.0-3 future_1.32.0 miniUI_0.1.1.1
[16] withr_2.5.0 spatstat.random_3.1-3 colorspace_2.0-3 progressr_0.13.0 knitr_1.39
[21] rstudioapi_0.13 Seurat_4.3.0.9002 ROCR_1.0-11 tensor_1.5 ggsignif_0.6.3
[26] listenv_0.9.0 labeling_0.4.2 bbmle_1.0.25 GenomeInfoDbData_1.2.4 polyclip_1.10-4
[31] bit64_4.0.5 farver_2.1.1 coda_0.19-4 parallelly_1.34.0 vctrs_0.4.1
[36] generics_0.1.3 xfun_0.31 R6_2.5.1 apeglm_1.12.0 rsvd_1.0.5
[41] locfit_1.5-9.4 spatstat.utils_3.0-1 bitops_1.0-7 cachem_1.0.7 DelayedArray_0.16.3
[46] assertthat_0.2.1 promises_1.2.0.1 scales_1.2.1 gtable_0.3.1 beachmat_2.6.4
[51] globals_0.16.2 goftest_1.2-3 rlang_1.0.2 genefilter_1.72.1 splines_4.0.3
[56] lazyeval_0.2.2 spatstat.geom_3.0-6 broom_0.8.0 BiocManager_1.30.15 yaml_2.3.7
[61] reshape2_1.4.4 abind_1.4-5 modelr_0.1.8 backports_1.4.1 httpuv_1.6.9
[66] tools_4.0.3 ellipsis_0.3.2 RColorBrewer_1.1-3 ggridges_0.5.4 Rcpp_1.0.8.3
[71] sparseMatrixStats_1.2.1 zlibbioc_1.36.0 RCurl_1.98-1.3 deldir_1.0-6 pbapply_1.7-0
[76] cowplot_1.1.1 zoo_1.8-10 SeuratObject_4.1.3 haven_2.5.0 cluster_2.1.0
[81] fs_1.6.1 magrittr_2.0.3 data.table_1.14.8 scattermore_0.8 lmtest_0.9-40
[86] reprex_2.0.1 RANN_2.6.1 mvtnorm_1.1-1 fitdistrplus_1.1-8 hms_1.1.1
[91] patchwork_1.1.2 mime_0.12 evaluate_0.20 xtable_1.8-4 XML_3.99-0.6
[96] emdbook_1.3.12 readxl_1.4.0 gridExtra_2.3 compiler_4.0.3 bdsmatrix_1.3-6
[101] KernSmooth_2.23-17 crayon_1.5.2 htmltools_0.5.4 later_1.3.0 tzdb_0.3.0
[106] geneplotter_1.68.0 lubridate_1.8.0 DBI_1.1.2 dbplyr_2.2.0 MASS_7.3-53
[111] Matrix_1.5-3 car_3.1-0 cli_3.3.0 igraph_1.3.0 pkgconfig_2.0.3
[116] numDeriv_2016.8-1.1 sp_1.5-0 spatstat.sparse_3.0-0 plotly_4.10.1 xml2_1.3.2
[121] annotate_1.68.0 dqrng_0.3.0 XVector_0.30.0 rvest_1.0.2 digest_0.6.29
[126] sctransform_0.3.5 RcppAnnoy_0.0.20 spatstat.data_3.0-0 rmarkdown_2.20 cellranger_1.1.0
[131] leiden_0.4.3 uwot_0.1.14 DelayedMatrixStats_1.12.3 shiny_1.7.4 nlme_3.1-149
[136] lifecycle_1.0.1 jsonlite_1.8.4 carData_3.0-5 viridisLite_0.4.1 fansi_1.0.3
[141] pillar_1.8.1 fastmap_1.1.1 httr_1.4.5 survival_3.2-7 glue_1.6.2
[146] png_0.1-8 bit_4.0.4 stringi_1.7.6 blob_1.2.3 BiocSingular_1.6.0
[151] memoise_2.0.1 irlba_2.3.5.1 future.apply_1.10.0
I have tried plotting that and I just get many zero values?
This also gives an unexpected volcano plot: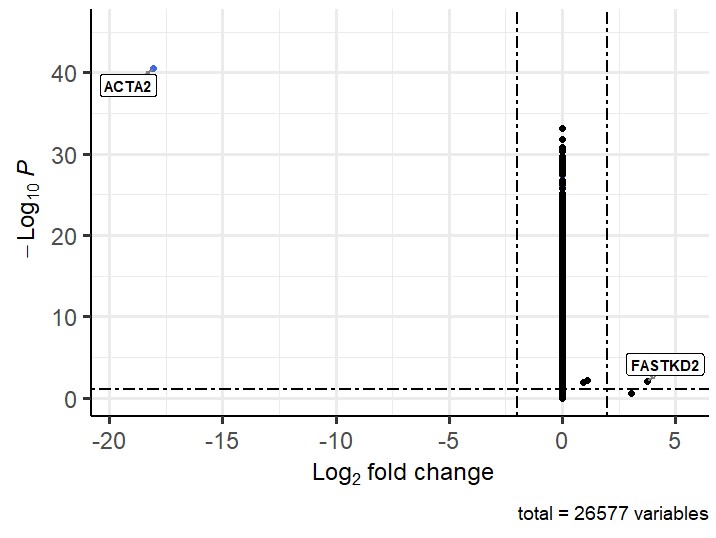
My guess is that the groups are too heterogenous to combine them and you should just run pairs, like so:
Hi Michael, thanks for your help with this.
I have run your suggestion above in combination with Lfcshrink and the log fold change values now seem sensible but the p values seem wrong. I have attached a volcano plot showing the problem. The cluster that is sitting centrally seems to be the cluster I previously had large negative fold change values for that was clustering on the left (which I still get if I dont perform lfcshrink). Any suggestion for the strange p-values? Thanks
Can you show code where you are getting the LFC and p-value? I'm suggesting to use the subsetting for both LFC and p-value estimation