Hi, I'm trying to assess differential expression in my total RNASeq data. I have several groups: Baseline, infected Pathogen1, infected pathogen 2, infected pathogen 1+2) where infected groups were sampled across 4 days. Pathogen 1+2 at 7 days has only 2 samples where the rest have 4 samples at each time (except baseline n=3). I'm planning to compare all of the groups (considering each time point from the infected groups as separate group) using a linear model to baseline.
I'm concerned about the plot of mean variance trend because it looks like I have not filtered the data sufficiently- the trend resembles a normal curve (ref: https://stats.stackexchange.com/questions/160255/voom-mean- variance-trend-plot-how-to-interpret-the-plot & Law et al., 2018). I'm not sure what the best approach would be to remedy this as suggested in Law et al. if I need to filter more.
Note: Filtering based on the group with the least amount of samples (2) and for cpm considered an avg of 5 million reads per sample (range: 27k - 10 million)
#1d. Create DGElist, d1
d0 <- DGEList(mouse.counts)
head(d0)
dim(d0) #134,071 x 49
## PREPROCESSING AND NORMALIZATION
# 2. FILTER genes that have CPM values above 2 in at least two libraries:
keep <- rowSums(cpm(d0) > 2) >= 2
d <- d0[keep, , keep.lib.sizes=FALSE]
dim(d) #29089 genes remaining
#Calculate norm factors after filtering.
dt <- calcNormFactors(d)
dt$samples
dgex2 <- estimateCommonDisp(dt, verbose=TRUE)
degxplot2 <- plotBCV(dgex2) #BCOV 3.7
#Look at the multidimensional scaling plot
#MDS plot colored by "Group' ordering: (color, #of samples corresponding to this color), cex is the font size, pairwise comparisons
plotMDS(dt, col=c(rep("black",3), rep("red",4), rep("red",4), rep("red",4), rep("red",2), rep("blue",4), rep("blue",4), rep("blue",4), rep("blue",4), rep("green",4), rep("green",4), rep("green",4), rep("green",4)), cex=0.3)
#common genes for each comparison
plotMDS(dt, col=c(rep("black",3), rep("red",4), rep("red",4), rep("red",4), rep("red",2), rep("blue",4), rep("blue",4), rep("blue",4), rep("blue",4), rep("green",4), rep("green",4), rep("green",4), rep("green",4)), cex=0.5, gene.selection = "common")
#Write the log-transformed normalized expression data (filtered data)
logcpm <- cpm(dt, prior.count=2, log=TRUE)
avelogcpm <- aveLogCPM(dt)
hist(avelogcpm)
##DESIGN MODEL MATRIX
group <- metadata1$Group #metadata table considers each tx & day as a separate group
group <- factor(group)
table(group)
Baseline.D0 IBV_Spn.D1 IBV_Spn.D3 IBV_Spn.D5 IBV_Spn.D7 IBV.D1 IBV.D3 IBV.D5
3 4 4 4 2 4 4 4
IBV.D7 Spn.D1 Spn.D3 Spn.D5 Spn.D7
4 4 4 4 4
mmg <- model.matrix(~group)
ydt <- voom(dt, mmg, plot = T) #norm factors calculated on filtered data
#test model fitting
#fitting linear models with Limma
fit <- lmFit(ydt, mmg)
head(coef(fit))
plotSA(fit)
#6b. Empirical Bayes smoothing of standard errors (shrinks standard errors that are much larger or smaller than those from other genes towards the average standard error) (see https://www.degruyter.com/doi/10.2202/1544-6115.1027)
efit <- eBayes(fit)
head(efit)
plotSA(efit)
sessionInfo( )
Gordon, thank for your response. 'filterByExpr()' was marginally better. I can see high variability in samples within the groups from the MDS plot. I tried fitting the model using weighting following Liu et al. 2015 (voomWithQualityWeights) to deal with this. I think that maybe using Voom with block weights might be best for helping with the variation or considering to discard some samples since I have 4 biological replicates in most groups. If you have any further suggestions or insights on handling the variation in this dataset, I would appreciate your input.
gene.selection=common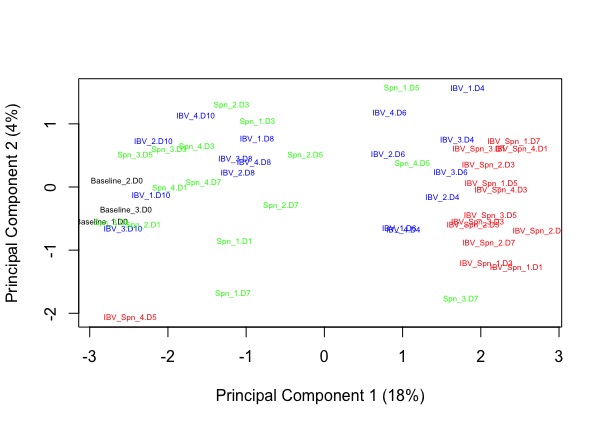